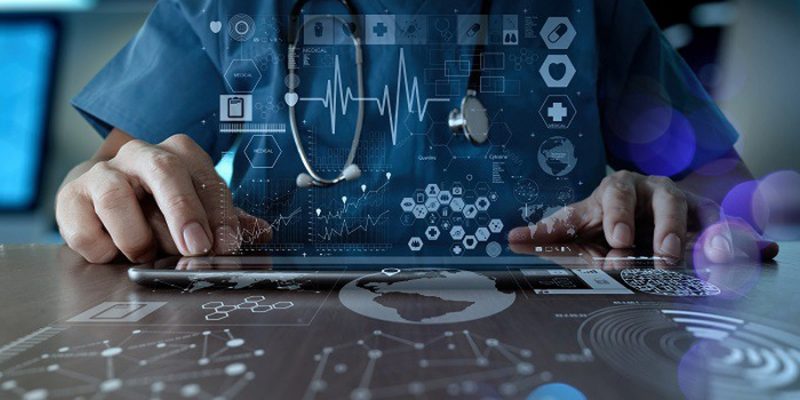
Doctors must be well acquainted with data science in 2022
In the biomedical research field, data science and artificial intelligence are no longer buzzwords. Hospitals and health insurance companies are increasingly encouraging physicians and other caregivers to use continuous data generated by wearable medical devices. Patients are supposed to undergo a full medical check-up once a month, rather than continuous monitoring of a handful of key metrics, in traditional healthcare, which mainly relies on high accuracy sparse datasets. Data science will have the greatest influence in assisting physicians in extracting therapeutically useful information from such massive, low-quality data sets.
To predict the risk of coronary artery disease from non-contrast chest CT images, radiologists and cardiologists are increasingly relying on automated high-dimensional image processing algorithms. Similar artificial intelligence-based technology is being used by radiologists and pulmonologists to determine clinically relevant structural and functional features of the lungs from chest CT images. Understanding the fundamentals of artificial intelligence will enable physicians to go beyond seeing these technologies as black boxes, allowing them to have the greatest influence on treatment routes.
The potential created by the marriage of data science and healthcare has already been seized by businesses. Omada Health, for example, created a programme focused on lowering the risk of avoidable health problems. It processes the patient’s behavioural data using data from smart devices such as scales and pedometers and then creates a highly tailored programme based on the results.
Another example comes from Enlitic, a company that links radiologists with data scientists to improve diagnosis accuracy and efficiency. Data scientists use deep learning algorithms to evaluate data from CT scans, X-rays, and other sources so that clinicians may “identify sooner with higher accuracy.”
We’ve outlined five reasons why physicians and caregivers should learn about new technologies like data science and artificial intelligence:
Large amounts of data obtained from continuous monitoring can be used to make diagnoses.
Wearable medical device firms such as CloudDX, Snap40, and QuasaR have enabled clinicians to examine continuous daily biometric data amassed over months. Using both primary and advanced data science methodologies, medically useful conclusions may be extracted from this rich data. Basic descriptive statistical measures, such as the patient’s average resting heart rate, can provide you with a quick image of their overall cardiac condition. More advanced indicators, such as the stress index or the LF/HF ratio of the RR distance, might be used to predict the likelihood of cardiac arrhythmias with more accuracy. On both local (days or weeks) and global (months or years) timescales, physicians will be able to examine these data sets using a combination of early warning ratings and visual assessment of the data utilising data science.
Diagnose using multiparameter data
In health care, merging several data sources typically yields the most meaningful insights. For example, total stress may be calculated by combining heart rate and heart rate variability. Internal as well as external causes, such as pollution, can promote respiratory disorders including COPD and asthma. Propeller Air, an open API that uses data from environmental sources to predict how asthma may be affected by local environmental conditions, combines patient respiratory health data collected using the Propeller spirometer with Propeller Air, an open API that uses data from environmental sources to predict how asthma may be affected by local environmental conditions. Learning data science techniques like data fusion might help doctors better comprehend how data is combined in these systems and, as a result, diagnose patients more quickly.
Diagnose using data visualization
Radiologists interpret high-resolution medical pictures like CT and MRI scans to help other experts like cardiologists and pulmonologists provide critical care. Radiologists are already employing machine learning-based software that colour codes the many aspects of an internal organ. Learning data science will assist radiologists in better grasping the software’s strengths and limits, allowing them to provide even better diagnostic results. Philips’ echocardiography, for example, makes use of an AI called HeartModelAI to create a 3D model of the patient’s heart using echocardiogram data. The FDA-approved Arterys AI-powered Cardiac MR Suite allows cardiologists to visualise the patient’s heart in 4D by colour coding the blood flow in the heart in real-time using magnetic resonance imaging (MRI) pictures.
Understand AI workflow
Physicians and other caregivers will soon encounter a variety of health predictors, such as early warning scores, that were created using deep learning. Cardiogram’s DeepHeart, for example, is a semi-supervised AI learning for cardiovascular risk prediction that works with Apple Watches. Understanding how these machine learning algorithms were created, as well as their limits, can assist caregivers in deciding how much to depend on these early warning scores.
Understand the statistical significance of clinical studies
Clinicians are continually learning about the newest and most fascinating case studies and clinical trials in their fields of expertise as part of continuing medical education. However, some of these findings may not be repeatable due to the small size of the patient group on which they were conducted. Learning data science can assist physicians in evaluating the importance of such research and deciding which ones to implement into their practice. Learning data science will also come in handy in the era of customised care when physicians will not only prescribe medication but also point out the likelihood of success depending on the patient’s genetic composition.