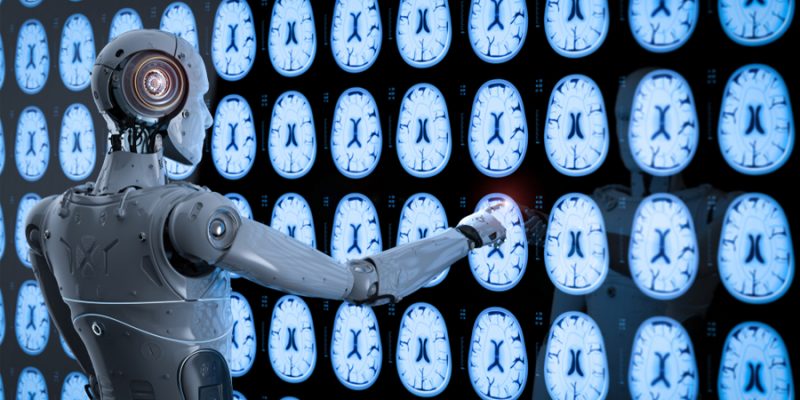
Medical imaging plays a crucial role in diagnosis and illness management. It enhances the effectiveness and value of healthcare services.
Professionals use large volumes of medical case data to train AI-based medical imaging algorithms to uncover patterns in pictures and identify particular anatomical biomarkers. The imaging algorithm may create metrics and outcomes to support the radiologist’s analysis by systematically evaluating the patterns of a digital picture, which is highly valuable for a speedy diagnosis. More than 100 high-resolution medical pictures can be processed with advanced medical imaging technology. Radiologists may now undertake inconspicuous evaluations and identify subtleties that are nearly hard to capture with the naked eye since the photos are incredibly quick.
It can accomplish quality productivity and accuracy in radiation workflow, as well as provide automatic prognostic information, which may be a valuable tool for identifying individuals at risk, in addition to establishing appropriate diagnostic testing in time to deliver improved health results. For them to obtain specialized and optimum medical care. Radiologists will not be replaced by AI-based medical imaging; rather, the number of radiologists providing clinical diagnosis and therapeutic decision support will increase, lowering the cost of medical mistakes and accidents.
Medical imaging is essential to healthcare because it allows doctors to detect disease early, better plan therapy, and improve patient care. Deep learning developments have demonstrated that computers can extract information from images more consistently and correctly than ever before, allowing clinicians to intervene early and better comprehend the whole illness process.
Machine learning and deep learning algorithms have proved their promise to enhance imaging diagnostics and patient care, from identifying lung cancer as well as human radiologists to forecasting kidney function from renal biopsies. The promise of AI, however, is not without its drawbacks. Patient safety risks, workflow interruptions, and accidental biases are all possible unintended effects of adopting the technology.
Develop standardized use cases
According to the organization, AI application cases in medical imaging have lacked consistent inputs and outputs among comparable techniques up until now. Because algorithms might operate on a local server or in the cloud, a common approach to receive and process inputs and outputs will be necessary. “It becomes difficult to generate standard data sets for training and testing without consistent inputs and outputs for AI use cases,” according to the paper, “and in the end, the resultant algorithms may produce various outcomes for the same discovery.” “Ideally, AI use cases should be written in a manner that turns human narrative explanations of what the algorithm should accomplish into machine-readable languages, such as Extensible Markup Language or JavaScript Object Notation, following well-defined rules.”
Establish data sharing methods
To develop high-performing AI algorithms, models must always be trained on elevated datasets with suitable annotations or rich metadata. Although there is a lot of activity in this area, the organization claims that it is largely focused on data-rich companies, which may restrict information availability. Privacy issues may limit institutions’ ability to make data public, limiting AI growth. According to the report, “accelerating the release of available to the public sets and Artificial intelligence methods like transfer learning that keep patient records behind an institution’s firewall while attempting to expose algorithm training too much larger datasets may well be able to help expedite the interpretation of AI in clinical practice.”
Assess clinical and infrastructure needs
The absence of user interfaces for integrating the outcomes of Algorithms into clinical processes, according to the paper, inhibits the adoption of AI models for wider clinical applications. To boost AI adoption, IT developers will need to provide effective usability and user experience that integrates with traditional medical workflow technologies. Additionally, developers would need to create supplier interoperability standards for health IT systems communication. “The medical imaging community must be active in analyzing clinical and infrastructural needs, as well as collaborate with existing standards organizations like the National Science Foundation and the NIH Connected Health Initiative to discover solutions that make AI adoption in clinical practice easier.”
Ensure technology is safe and accurate
Medical stakeholders should collaborate with IT programmers, government entities, and the general public to ensure that AI applications are accurate, devoid of prejudice, and healthy for patients, according to the organization. Stakeholders will need to evaluate AI algorithms utilizing datasets with a wide range of technological and market diversity to do so. The FDA, for example, has played a crucial role in verifying AI models to assure patient safety. “The FDA controls a wide range of medical imaging devices, as well as computer-aided diagnostic programs and other algorithms that assist medical practitioners in making decisions,” according to the article. “The agency understands the fast development in digitalization across the health care continuum and the significance of regulating software that may identify and categorize infections,” says the statement.