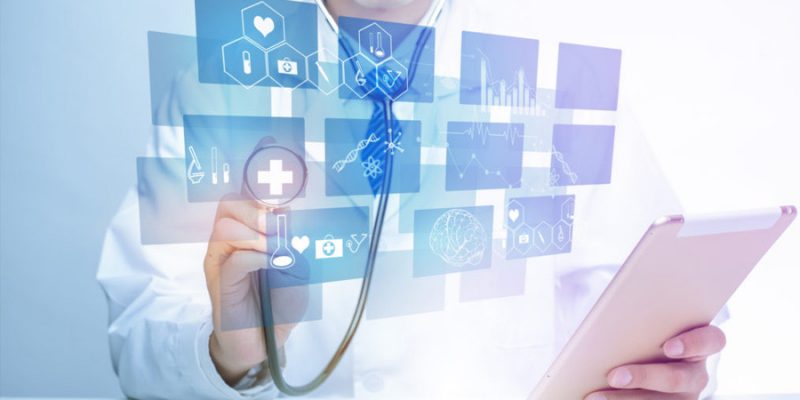
AI in healthcare is showing various improvements with AI algorithms and systems.
Artificial Intelligence(AI) is an effective tool that can help solve some of the most important challenges dealing with the healthcare sector nowadays while also ensuring the best patient care, decreasing fees, and lowering the potential liability for practitioners. Administrative tasks inclusive of information retention and maintenance, pre-authorizing coverage, and bill processing and follow-up absorb a significant part of the time that would otherwise be allotted to patient care. With the use of Artificial Intelligence systems, inclusive of a superior AI billing software program that automates the claims evaluation method, those administrative burdens are decreased. Another use of AI in the healthcare industry surrounds data analytics. Data analytics may be damaged down into 4 wonderful categories — descriptive, diagnostic, prescriptive, and predictive — all of which examine each modern and historic data to expect certain results as they relate to an individual. These analyses make use of additionally expanding to the population at massive to expect destiny health-related results by assessing broad, influential, community-associated elements similar to the modern and historic data. Data analytics is likewise used in patient care. For example, data analytics and clinical choice aid software are used to decorate the decision-making method by presenting remedy tips based on patient data, reference materials, medical guidelines, and medical journals. This in turn enables pick out the character of the clinical condition, and which sufferers are vulnerable to falls, hospital readmissions, and different healthcare conditions that can require intervention.
Additionally, data analytics and image-reputation software program are used to pick out arrhythmias in EKGs, or even come across malignant nodules in CT scans. Regardless of whether the makes use of AI in the healthcare sector could bring about optimized staffing, improved fee management, extended performance because it pertains to debts receivables and invoice processing, and decreased burnout, moral concerns should still be considered to make sure the quality of patient care.
While AI gives some possible benefits, there are also numerous risks:
The nirvana fallacy: One very last threat bears mention. Artificial intelligence can be excellent and suitable in healthcare. The nirvana fallacy posits that issues get up while policymakers and others evaluate a new choice to perfection, in place of the status quo. Health-care AI faces dangers and challenges. But the current device is likewise rife with issues. Doing nothing due to the fact AI is imperfect creates the threat of perpetuating a problematic status quo.
Injuries and mistakes: The maximum obvious risk is that AI systems will every so often be wrong, and that patient damage or different healthcare problems can also additionally result. If an AI system recommends the incorrect drug for a patient, fails to be aware of a tumor on a radiological scan, or allocates a hospital bed to 1 patient over some other as it is expected wrongly which patient might benefit more, the patient can be injured. Of course, many accidents arise because of clinical mistakes in healthcare devices today, even without the involvement of AI. AI errors are potentially different for at least one reason. First, sufferers and carriers may react in a different way to injuries as a consequence of software programs than from human mistakes. Second, if AI structures emerge as large, an underlying hassle in a single AI device would possibly bring about accidents to hundreds of sufferers—rather than the limited quantity of patients injured by any single provider’s error.
Privacy concerns: Another set of dangers gets up around privacy. The requirement of massive datasets creates incentives for builders to accumulate such records from many patients. Some sufferers can be involved and this series can also additionally violate their privacy, and lawsuits had been filed based on data-sharing among massive fitness structures and AI developers. AI ought to implicate privacy in some other way: AI can predict private facts about patients even though the algorithm in no way received those facts. (Indeed, that is frequently the aim of healthcare AI.) For instance, an Artificial Intelligence device is probably capable of perceiving that someone has Parkinson’s ailment based on the trembling of a computer mouse, even supposing the person had never revealed that fact to all and sundry else (or did not recognize it). Patients would possibly recall this as a contravention of their privacy, specifically if the AI system’s inference had been to be had to third parties, such as banks or life coverage companies.
Data availability: Training in Artificial Intelligence systems calls for large quantities of data from sources together with electronic health records, pharmacy records, coverage claims records, or consumer-generated information like health trackers or purchasing history. But health data are frequently elaborate. Data are usually fragmented throughout many different systems. Even other than the range simply mentioned, patients usually see different providers and transfer insurance companies, main to records cut up in a couple of structures and multiple formats. This fragmentation will increase the threat of mistakes, decrease the comprehensiveness of datasets, and will increase the price of collecting records—which additionally limits the forms of entities that can broaden powerful healthcare Artificial Intelligence.
Bias and inequality: There are risks related to bias and inequality in fitness-care AI. AI systems analyze the records on which they’re trained, and they could include biases from the one’s records. For instance, if the data to be had for AI are mainly amassed in instructional clinical centers, the ensuing AI systems will know much less about—and consequently will deal with less effectively—patients from populations that don’t typically have common academic medical centers. Similarly, if speech-recognition AI systems are used to transcribe encounter notes, such Artificial Intelligence can also additionally carry out worse while the issuer is of a race or gender underrepresented in training data.
Professional realignment: Longer-time period risks contain shifts in the clinical profession. Some clinical specialties, together with radiology, are likely to shift considerably as much of their work will become automatable. Some students are concerned that the large use of AI will bring about reduced human information and capability over time, such that carriers lose the capacity to catch and correct Artificial Intelligence mistakes and in addition to developing medical information.