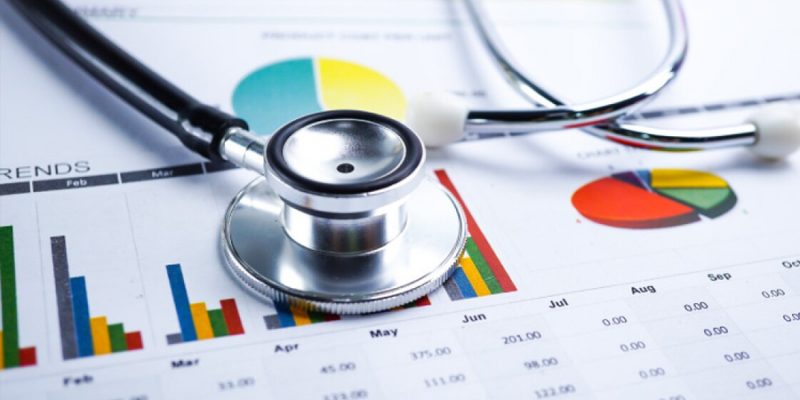
How predictive analytics is boosting the healthcare industry to perform more effectively, cost-effective, and reduce waste.
Artificial Intelligence is beneficial to every aspect of our lives. And one such aspect is an aspect of healthcare. With rising numbers of diseases and patients, early predictions can be lifesavers. Predictive analytics in healthcare refers to the analysis of current and historical healthcare data allowing healthcare professionals to derive effective and efficient operational and clinical decisions, predict trends, and manage the spread of diseases. Predictive analytics benefits three major areas including clinical care, administrative tasks, and operational management. Predictive analytics is beneficial to a multitude of sectors in healthcare. It can range from a private physician to a multispecialty hospital along with health insurance companies.
As of now, Electronic health records (EHR) in the healthcare industry provide predictive analytics capabilities. Users also hope for further additional capabilities. Healthcare IT providers also build their own analytics models to cater to optimal healthcare. They also collaborate with institutions to build advanced algorithms, improve clinical care, better administrative performance, and efficient operations. Such advancements are not novel in nature. The healthcare industry has been improving upon them in recent years. Some common applications are given below.
Prediction of conditions:
physicians, healthcare institutions, and insurance providers use predictive analytics to enunciate the likelihood of their patients developing certain medical conditions. By using national data, EHR data, biometric data, and claims data models are created. These models can later be used on a national or community or even individual level to predict any medical condition.
Understanding disease progression and comorbidities.
Predictive analytics in healthcare is also used to identify patients whose conditions can worsen. It is also used to identify the co-morbid conditions of the patients. Such identification is lifesaving. As these conditions are predicted at an early stage, the conditions are improved drastically. Such early predictions are an important capability to save lives.
Overstays at Hospitals:
By analyzing the clinical and departmental data, predictive analytics help in identifying patients who are more likely to exceed their stays. Such insights are useful for physicians. Physicians can adjust care protocols to keep patients’ recovery on track. These early predictions of overstays and the change in care protocols help patients to avoid overstays. By avoiding overstays patients are able to reduce expenses, not deplete hospital resources, and reduce the chances of secondary infections.
Readmission to hospital:
This is another prevalent use of predictive analytics in healthcare. By forecasting which patients are likely to get readmission to the hospital, clinicians are able to focus on post-hospitalization admissions. This small step enables patients to cut out the costs of readmission, save hospital resources and overall improve their outcomes.
Proper allocations of resources:
Healthcare organizations are large in size, scope, and complexities. Such a vast level hinders the effective and efficient allocation of resources. Predictive analytics identify patterns and future needs for resources. These predictive results will ensure the right amount of resources at the right time and to the right patient. This also prevents the wastage of resources.
Acquiring resources:
Based on data points like seasonal needs, demographic changes, and patient populations, healthcare organizations use predictive analytics to identify which additional resources are required. Predictive analytics is also helpful in identifying the need for machines or tools and resources associated with those. It also specifies the number of resources required for the anticipated caseload.
Managing supply chain:
Using predictive tools healthcare organizations can better manage their supply chains. By doing so, organizations can gain visibility about their vast and intricate supply requirements. This will further help in vendor consolidations and streamlined purchases. Thus, organizations are performing more efficiently, saving costs and reducing waste.
Patient engagement and behavior:
Predictive analytics pave the way for the subjective understanding of patients. This aids in providing treatment plans curated solely for a patient and predicting which patient is more likely to adhere to their treatment plans and who won’t. By understanding each patient and their subjective needs and preferences healthcare organizations can work more effectively and provide better healthcare outcomes.
Consumer choice:
A thorough understanding of patients’ subjective needs and preferences will not only allow customized treatment plans but also help them pick a clinician who is just right for them. Predictive analytics analyzes the patient’s specific requirements and offers a list of providers, even in case of emergencies.
Refunding of Insurance:
Predictive analytics allows for the optimization of financial performance. By thorough analysis, healthcare organizations can identify documents that are most likely to be declined and documents that would provide a higher yield. Predictive analytics looks for missing or insufficient refunds as well as identifies opportunities to upcode.