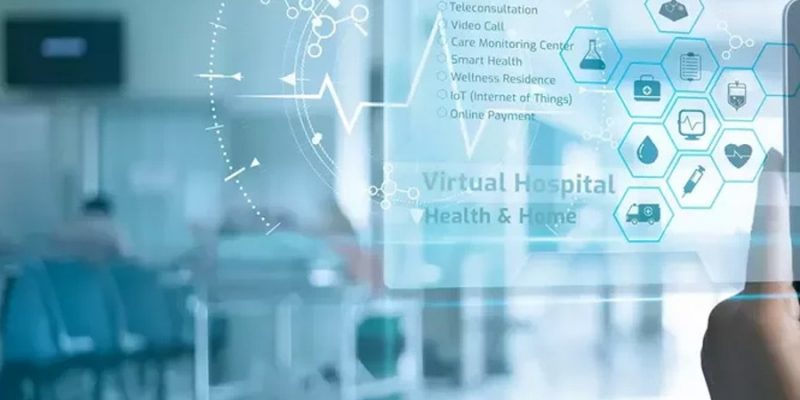
Healthcare providers are significantly impacted by financial incentives for services that Artificial Intelligence would help.
Artificial intelligence (AI) technologies that benefit patients and populations must be financially motivated at a constant and sustainable level for their use in healthcare to be adopted responsibly. However, AI that does not provide these health advantages should not be rewarded. We concentrate on patient-specific, helpful, and autonomous AI systems, where such financial incentives are supervised by both public and private payors and also necessitate participation, oversight, and support from affected stakeholders in healthcare, such as patients, policymakers, clinicians, regulators, provider organizations, bioethicists, and AI developers. According to an early study by Abramoff and other researchers, such assistance and participation were requested, which resulted in the development of an ethical framework for healthcare AI.
Healthcare providers are significantly impacted by financial incentives for services that the AI would help with when deciding whether to acquire and use an AI tool, the authors claim. The adoption of AI is significantly influenced by several factors, including compensation and insurance coverage. Currently, one FDA-approved autonomous AI system has a nationwide payment plan set up by the Centers for Medicare and Medicaid Services (CMS). Under the New Technology Add-on Payments (NTAP) framework, the agency has also developed a national add-on payment for assistive AI.
According to the researchers, NTAP has resulted in CMS reimbursements for AI, but these payments are severely constrained due to a variety of variables, including the fact that the program is technology-specific, has a convoluted approval process, and only covers inpatient services. As a result, other researchers have made an effort to create new healthcare AI frameworks, but according to the authors of the report, these frameworks fail to take into account the sometimes intricate US healthcare coverage and reimbursement systems. They also fail to consider the roles of those affected, like patients, providers, lawmakers, payers, and AI developers.
The authors instead suggest their framework, which is intended to be open, maximizes alignment with ethical frameworks for healthcare AI, permits more optimal alignment between the ethical, equity, workflow, cost, and value perspectives for AI services, and increases support from impacted stakeholders and map onto the current payment and coverage systems in the US. The authors contend that they are crucial to ensuring healthcare quality, and healthcare equity, and mitigating possible bias in businesses implementing AI.
The researchers used a case study for an autonomous AI system to demonstrate how their methodology may be applied to current regulatory, care, and payment systems. AI is frequently used in clinical settings to identify diabetic retinopathy and diabetic macular degeneration.
According to the authors, the tool’s developer costs $55 for this AI service, the AI labor, and receives AI inputs and outputs for each patient. The researchers contend that in doing so, the designer employs “access maximizing value,” a definition of value that the authors apply in their framework to reduce spending per patient while encouraging service access.
A more expensive retinal camera gear, for instance, would have allowed for improved accuracy and diagnosability at a lower expense for the diagnostic AI algorithms, but this would raise the cost per patient. In contrast, a cheaper camera would need more complex, pricey AI algorithms, yet the developer can still decide to charge $55 because AI algorithms are scalable, enabling the developer to maybe split the additional expenses over many patients.
The authors’ approach also mentions several “guardrails” and compensation methods that are supervised by stakeholders as a way to assist enforce moral standards in the application and usage of AI. For instance, the American Diabetes Association and the FDA both approved the device, the CMS developed a national payment plan for the device, and commercial insurance companies utilized the CMS rate, among other things, to determine their payment levels.
According to the authors, each stakeholder’s actions led to a balance between the ethical, workflow, financial, and value aspects of AI in healthcare. The researchers claim that similar evaluations utilizing their framework can direct the development of future AI applications and capabilities.
Analytics powered by AI to help decision-making
To quickly identify COVID-19, researchers at the Mount Sinai health system have built an AI algorithm that combines patients’ chest CT scans with clinical data such as symptoms, age, blood reports, and potential contact with infected individuals. Using distinct probabilities of CT scans, clinical data, and both combined, the program gives a final analysis and replicates the physician procedure used to detect COVID-19. Additionally, 68 percent of COVID-19-positive patients were found by the AI system in cases where radiologists mistook them for negative ones because of the negative CT look. Infected patients can be evaluated more quickly in the emergency room by using the system to triage or prioritize them early on in their admission. It can also be used to provide doctors with a second perspective.
Encouraging scientific discovery
Imagine, an AI healthcare business has partnered with US and Canadian institutions to use its EVIDENCE platform to speed up the process of scientific discovery. By providing automatic data segmentation and labeling, the platform gives doctors the ability to organize data from real-time hospital systems. It scales up conventional scientific discovery procedures by converting unstructured clinical patient data into structured information based on outcomes. Through the use of the EVIDENCE platform, hospitals are collaborating to analyze treatments and outcomes to improve medical outcomes for patients with lung cancer.
Since the start of the pandemic, scheduling and organizing clinical staff rotations has been a significant concern for health systems. AI-powered solutions can help by taking into account operational restrictions including the quantity of personnel, availability, capabilities, and necessary equipment.